Suicide remains a major public health crisis, claiming approximately 14.2 lives per 100,000 Americans each year. Despite the prevalence of suicide, many people who die by suicide have interactions with health care providers in the year before death, and the reasons for this are often unrelated to mental health. .
This highlights critical gaps in routine risk identification and the need for innovative solutions to strengthen suicide prevention efforts.
A recent study conducted by researchers at Vanderbilt University Medical Center provides promising insights into how artificial intelligence (AI) can fill this gap.
The study, published in the journal JAMA Network Open, was developed by Vanderbilt Suicide, an AI system designed to analyze routine data from electronic health records (EHRs) to calculate a patient’s 30-day suicide risk. Focused on the Attempt and Ideation Possibility Model (VSAIL). . This study aimed to improve suicide risk assessment during routine medical visits, particularly in neurology clinics, by leveraging an AI-driven clinical decision support (CDS) system.
The study was a randomized controlled trial (RCT) that enrolled 7,732 patients over a six-month period at three Vanderbilt neurology clinics. This study compares two CDS approaches: disruptive alerts, which actively interrupt a clinician’s workflow to facilitate suicide risk assessment, and non-disruptive alerts, which passively display risk information in the patient’s electronic health record. I did.
These clinics were selected because of their patient populations, including patients with neurological conditions associated with higher suicide risk, such as Huntington’s disease and certain movement disorders.
The researchers hypothesized that interruption alerts would be more effective in encouraging in-person suicide testing. The primary objective of this study was to assess whether interrupted CDS leads to higher screening rates compared with non-disruptive CDS. A secondary objective investigated how these alerts compared to previous year testing rates.
The research team’s focus on neurology clinics was strategic. Unlike high-risk settings such as emergency departments, these clinics do not have universal screening protocols. However, certain neurological disorders are associated with increased suicide risk, highlighting the need for targeted interventions in these settings. This trial is one of the first attempts to evaluate suicide prevention CDS in a randomized clinical framework.
The results of this study highlight the potential of AI-driven CDS systems to enhance suicide prevention in medical settings. Disruptive alerts led to suicide risk assessments in 42% of flagged visits, significantly outperforming non-disruptive systems that prompted screening in just 4% of cases.
Although the automated system flagged approximately 8% of all patient visits, its selective nature was considered feasible for implementation in a busy clinical environment.
Dr. Colin Walsh, lead author of the study and associate professor of biomedical informatics, medicine, and psychiatry, emphasized the importance of targeted interventions. “Universal screening is not practical in every situation,” Walsh explained. “We developed VSAIL to identify high-risk patients and encourage intensive screening conversations.”
Despite the effectiveness of interruptive alerts, this study acknowledged potential downsides, such as alert fatigue, where clinicians can be overwhelmed by frequent notifications. Future research is needed to balance the benefits of these alerts with their impact on workflow. “Healthcare systems need to balance the effectiveness of interruption alerts with the potential downside,” Walsh added.
Suicide risk screening has traditionally relied on clinical judgment and validated instruments such as the Patient Health Questionnaire and the Columbia Suicide Severity Rating Scale.
However, gaps in reliable screening still exist, especially in non-mental health settings. Research shows that 77% of people who die by suicide see a primary care provider in the year before death, highlighting the importance of improving risk identification in these encounters.
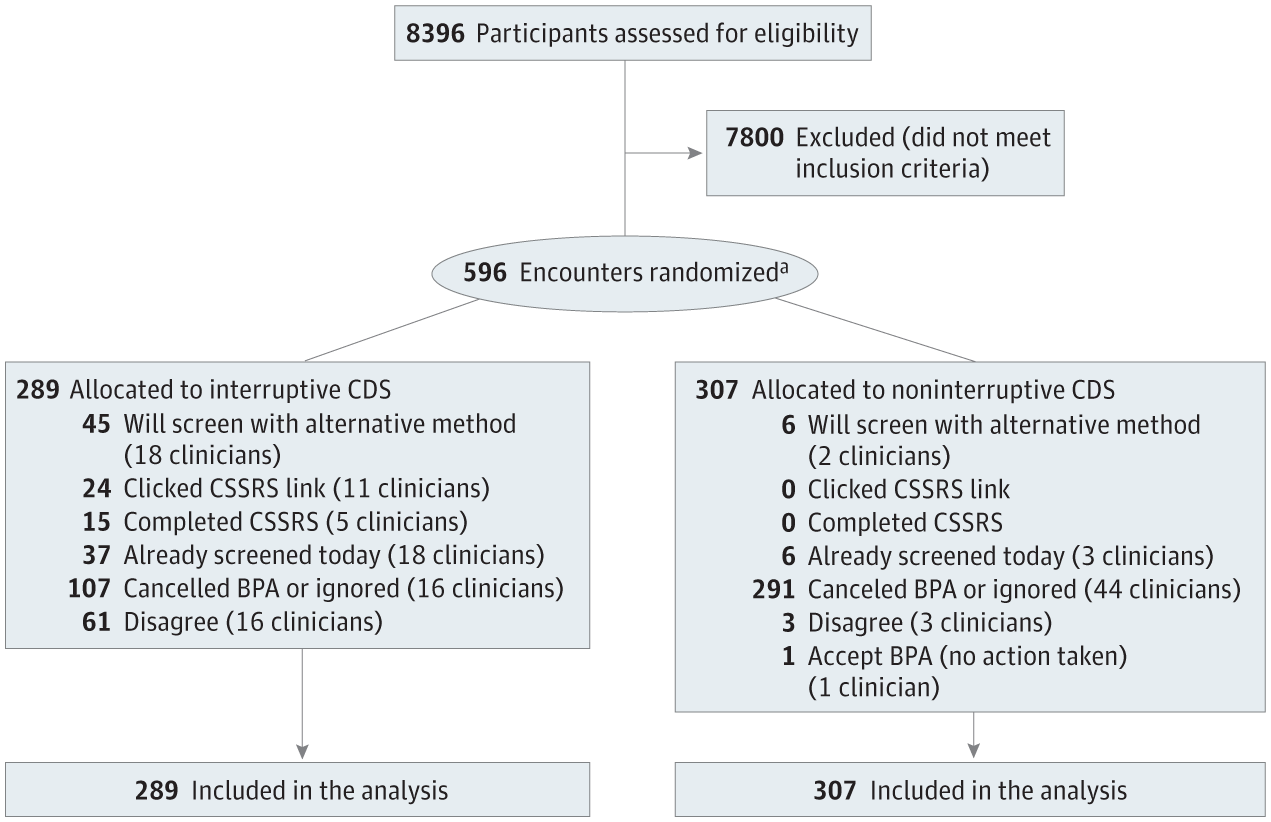
The VSAIL model represents a shift toward computational risk estimation that can complement traditional methods. This study demonstrated that by integrating predictive modeling into EHR systems, AI can enhance clinicians’ ability to identify and assess at-risk patients.
Previous testing of the model, which ran silently in the background without triggering alerts, confirmed its accuracy in identifying high-risk individuals. Of the flagged patients, 1 in 23 later reported suicidal ideation.
Although this study focuses primarily on neurology clinics, its implications extend to other medical settings. For example, primary care remains an important point of contact for individuals at risk of suicide. With 77% of suicide victims visiting a primary care provider in the year before death, implementing AI-driven CDS in these settings could significantly enhance prevention efforts.
The researchers also noted that the model’s selective approach, which flags just 8% of patient visits, made it more practical for busy clinics. This selective process ensures that clinicians are not overwhelmed with alerts and can focus on high-risk individuals without sacrificing quality of care.
The results of this study have significant implications for suicide prevention efforts in a variety of medical settings. Although the study focused on neurology clinics, the researchers suggested that similar AI-driven systems could be tested in primary care and other specialties. Expanding the application of such models could help address the broader challenge of timely suicide risk assessment.
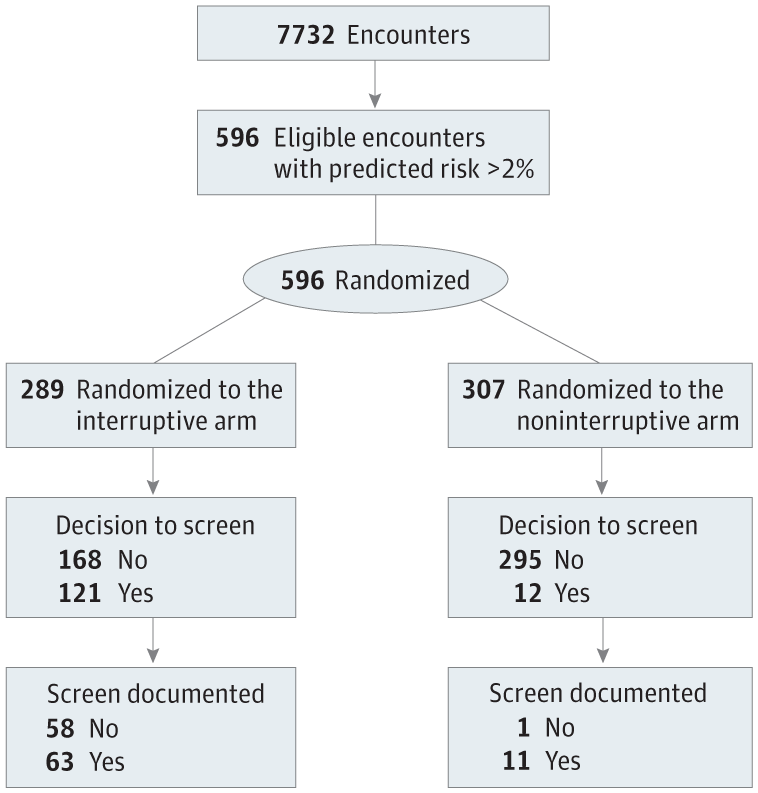
During the study’s 30-day follow-up period, no patients flagged by either alert group experienced suicidal thoughts or attempts. Although the results are encouraging, the researchers caution against complacency and highlight the need for continuous evaluation of CDS systems to ensure their effectiveness and minimize unintended consequences. emphasized.
This study also highlighted the importance of human-centered design in the development of CDS systems. In this study, we aimed to create an effective and minimally disruptive tool by tailoring alerts to clinicians’ workflows and taking their feedback into account.
“The automated system only flagged about 8% of all patients who came in for screening,” Walsh said. “This selective approach makes it more feasible for busy clinics to implement suicide prevention efforts.” You’ll be able to focus on meaningful interactions.
Additionally, the researchers emphasized the need to address vigilance fatigue in future studies. Disruptive alerts have been found to be more effective, but excessive notifications can weaken their impact over time. Future research should consider strategies to alleviate this problem so that alerts remain effective without burdening healthcare providers.
This research also provides a framework for integrating AI-driven tools into existing healthcare systems. By leveraging predictive models like VSAIL, healthcare professionals can enhance their ability to identify and support at-risk patients. These advances could play a vital role in reducing suicide rates and improving overall patient outcomes.
As suicide rates continue to rise, innovative approaches like the VSAIL model offer a promising path to strengthen prevention efforts. Integrating AI-driven tools into routine medical practice will enable clinicians to more effectively identify and support at-risk patients.
Although challenges such as vigilance fatigue remain, the findings highlight the technology’s potential to transform suicide prevention strategies in healthcare settings. With further research and refinement, these systems could play a vital role in reducing suicide rates and saving lives.