We shape the future of AI-powered business by providing secure, high-performance, interconnected infrastructure
Based on previous articles, AI innovations will not slow down. How multi-model AI is delivering speed continues in its thought leadership series in AI.
Digital Realty closely tracks AI evolution between hardware, software and networking, providing businesses with meaningful insights that help them efficiently scale private AI. As AI adoption grows, businesses need to concentrate on security, optimization, and interconnection to integrate private data with public AI models.
This article covers three key areas that companies need to address in order to enable private AI at scale.
Enabling Private Data for Public AI Models: Private Data Ecuring ASECASING ASECURING PRIVATE FREAMWORKS and Compliance Considerations How businesses can efficiently integrate key security frameworks and compliance considerations to protect data centers and interconnected fabrics: Workload placement, data centers, AI success
Enabling private data for public AI models
The next wave of AI innovation is not just about bigger models – it’s about smarter infrastructure
Chris Sharp, Digital Realty
Integrating private datasets with public AI models requires a balance of accessibility and security. Companies need to ensure the interaction of structured data access and controlled AI models to maximize performance while maintaining privacy. The important steps are:
Hybrid approaches that utilize frontier models such as GPT, Claude, and Gemini enable a wide range of AI inference capabilities from generalized models along with industry-specific AI, enhancing accuracy from custom-trained industry AI solutions. This method improves performance through strategic workload distribution across cloud, on-premises, and edge environments.
For example, large financial institutions such as JPMorgan Chase leverage private AI models to optimize risk assessment, fraud detection and customer service. By integrating their own financial datasets with AI, these companies will strengthen their decision-making and ensure compliance with strict regulatory requirements.
Similar approaches can be adopted in other industries, such as healthcare and manufacturing, to drive specialized AI applications beyond the capabilities of general-purpose models.
Protect your private data with AI workflows
To keep private data secure with AI workflows, you need a zero trust model. This assumes that a user or system should not be inherently trusted, regardless of location or credentials. Companies need to take all of the approaches to prevent unauthorized access and data breaches.
By incorporating zero trust principles such as end-to-end encryption into AI workflows, businesses can protect sensitive data, reduce exposure risk, and maintain compliance while scaling AI adoption.
Companies need to implement AI safety guardrails and model lifecycle security to ensure that the model works as intended and remain secure from development through deployment and continuous operation. The key components are:
Input validation: Preventing AI models from handling malicious or biased data inputs handle data inputs that can lead to false or unethical decisions. Enforce security without performance trade-offs. The following criteria, such as Miter Atlas (Adversarial Threat Landscapes of Artificial Intelligence Systems) and NIST (National Institute of Standards and Technology), ensure strong defenses, especially as agent AI workflows dynamically divide tasks across multiple models.
– Getty
Optimize AI performance with data centers and interconnect fabrics
Having a powerful model isn’t just about making AI the best possible performance. It involves putting your workloads in the right environment, ensuring fast connectivity and managing costs. Companies need a combination of cloud, edge and private data center deployments to achieve the right balance between performance, security and efficiency.
Strategic workload deployment improves AI performance while managing costs.
AI workload types, optimal deployment, key benefits training, cloud AI clusters, scalable computing power batch inference, private data centers, security and cost-effective real-time inference, edge AI deployments, and low latency respiration, and low latency-less pole lag (total search generation) query processing, AI exchanges, and disparity spreading data moves seamlessly between locations. To maintain AI responsiveness and efficient interconnection fabrics (such as Digital Realty Services Fabric), fast, low latency connectivity is essential to easily synchronize AI workloads in Clud, Edge, and Enterprise environments, such as private AI exchanges in Digital Realty, to enable businesses to access and ensure public models between external AI models. Allows businesses to connect AI applications across multiple cloud providers without vendor lock-in
As AI adoption accelerates, inference workloads require low latency, high bandwidth environments near dense metropolitan areas. Digital Realty’s core market infrastructure bridge cloud, edge and enterprise enable AI models to run efficiently in close proximity to data sources and users.
The future of AI is interrelated
The next wave of AI innovation is not just about bigger models, but smarter infrastructure. Companies need to focus on seamless data integration, security, and optimized performance to unlock the possibilities of AI completely.
Digital Realty doesn’t just support AI adoption. By providing safety, high performance, and interconnected infrastructure, it shapes the future of AI-powered business. Whether you’re scaling private AI, securing data, or optimizing workloads, there are solutions to help you.
Get started with today’s Digital Realty and start building your AI-Reaid infrastructure.
From Digital Realty
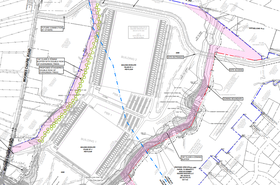
April 28, 2025
The company is also acquiring land to expand existing campuses
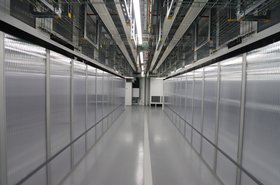
April 8, 2025
HER1 consists of prefabricated Schneider Electric modules
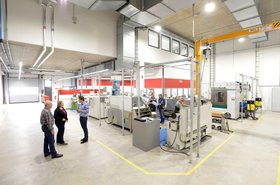
April 3, 2025
Heat is delivered from the FRA14 data center